
Benefits of predictive analytics for marketing
Table of contents
Quick Access
Predictive analytics is one of the approaches or solutions generated from data analytics, which, as the name implies, assists organizations or marketing teams in anticipating what the client or public is thinking or expecting to acquire.
"Predictive analytics refers to the use of statistics and modeling techniques to forecast future outcomes and performance." Predictive analytics examines current and past data patterns to see if they are likely to reappear. This enables businesses and investors to reallocate their resources in order to capitalize on potential future events. "Predictive analytics can also be used to improve operational efficiency and reduce risk," Investopedia explains.
The significance of client behavior in marketing
When creating an advertising campaign or considering a commercial for a product or service, the first thing a marketing team considers is the client's needs: how to fit the advertising to what the public wants and thus ensure that it is as effective as possible.
On rare circumstances, the client's needs change; this can happen gradually over time or suddenly; if this occurs, the entire campaign and the considerable work put into it will be for naught. Predictive analytics can help to avoid this.
How to Predict Customer Behavior Using Predictive Analytics
Predictive analytics is a strong tool that can assist businesses in anticipating customer behavior and making sound decisions. Here are the steps to using predictive analytics to anticipate client behavior effectively:
Establish your objectives
Define the precise client behavior you wish to anticipate. Predicting purchasing trends, churn, client lifetime value, or any other important activity could be included.
Gather information
Collect relevant data on your clients, such as demographics, transaction history, website interactions, social media activity, customer service interactions, and any other information that is relevant. Check that your data is correct, full, and well-organized.
Data cleaning and preprocessing
Accurate predictions require high-quality data. Remove duplicates, repair errors, and handle missing numbers to clean up data. Preprocess data by putting it into a predictive analysis-ready format, such as normalizing numeric data or coding categorical variables.
Choose the best forecasting models
Based on your aims and the type of your data, select the appropriate predictive modeling tools. Regression analysis, decision trees, random forests, neural networks, and clustering algorithms are all often utilized techniques.
Develop the predictive model
Divide your data into training and testing sets. Build and train your predictive model using the training data. Patterns and correlations between consumer qualities and behaviors must be learned by the model.
Validation and fitting of the model
Using the test data set, evaluate the performance of your predictive model. To evaluate your performance, use metrics such as accuracy, precision, recall, or area under the curve (AUC). Tune the model by tweaking the parameters or experimenting with other algorithms as needed.
Make forecasts
Once the model has been tested and proven to be accurate, use it to generate predictions on fresh and previously unseen data. This could include assessing real-time data as it becomes available or applying the model to historical data to forecast future behavior.
Predictions should be interpreted and acted upon
Analyze your model's predictions to acquire insight into customer behavior. Determine the patterns, trends, and factors influencing customer behavior. Make informed company decisions and take proactive efforts to engage customers, boost customer happiness, and optimize marketing campaigns using this information.
Maintain and update
Monitor the success of your prediction model over time on a regular basis. Update your model as client behavior changes to ensure its accuracy and relevance. To enhance the success of your predictive analytics approach, analyze and refine it on a regular basis.
Remember that predictive analytics is an iterative process and requires constant refinement and improvement. By harnessing the power of predictive analytics, you can gain valuable insight into customer behavior and stay one step ahead of meeting their needs and expectations.
We recommend you on video
Related blogs
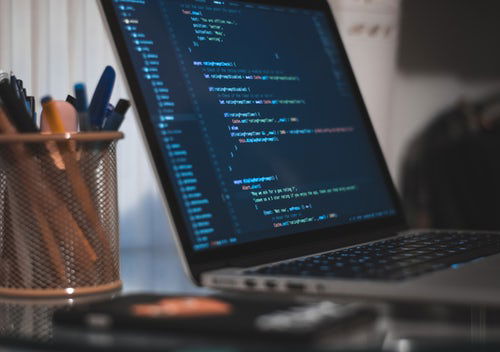
5 Tips for outsourcing QA in Costa Rica

How to implement Open Banking in Colombia?
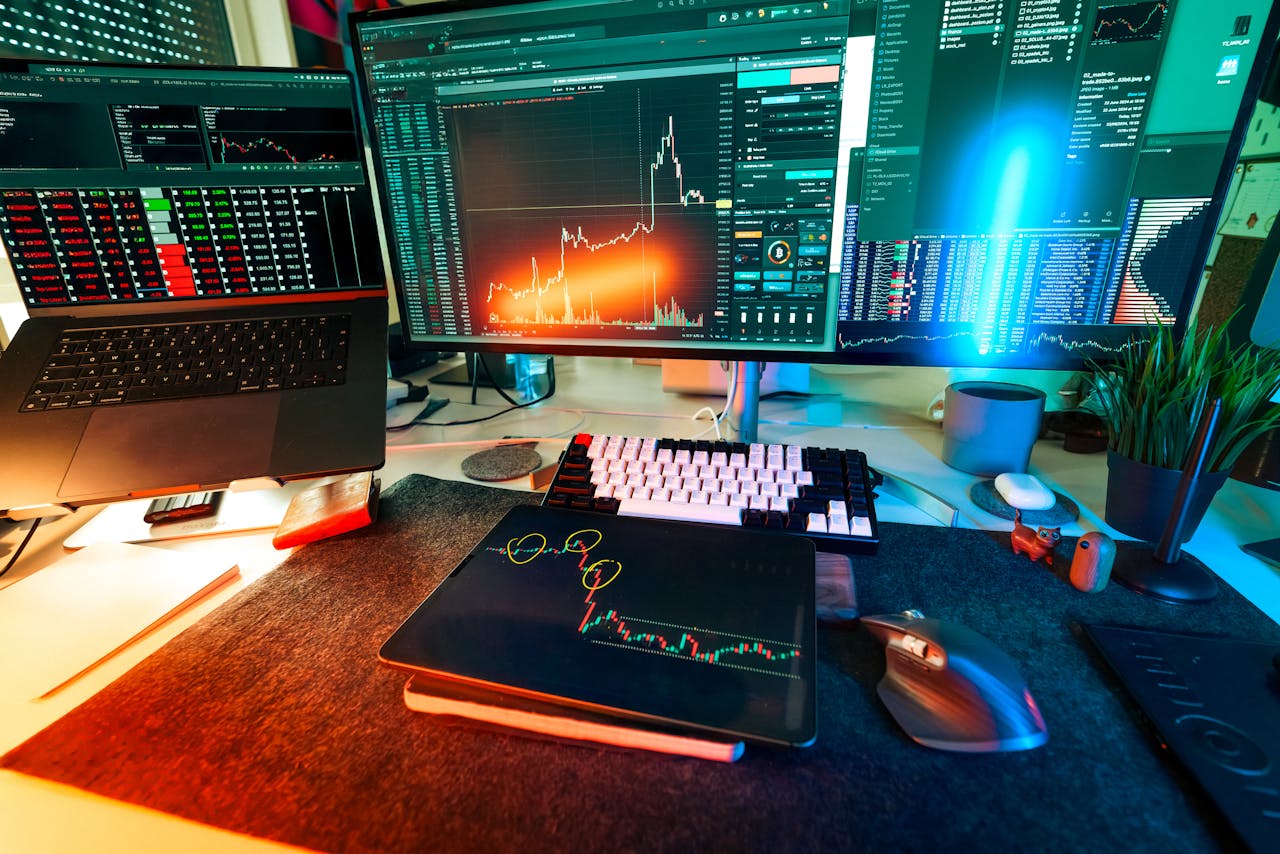
API payment integration service: How do they work?
Top fintech software development company services
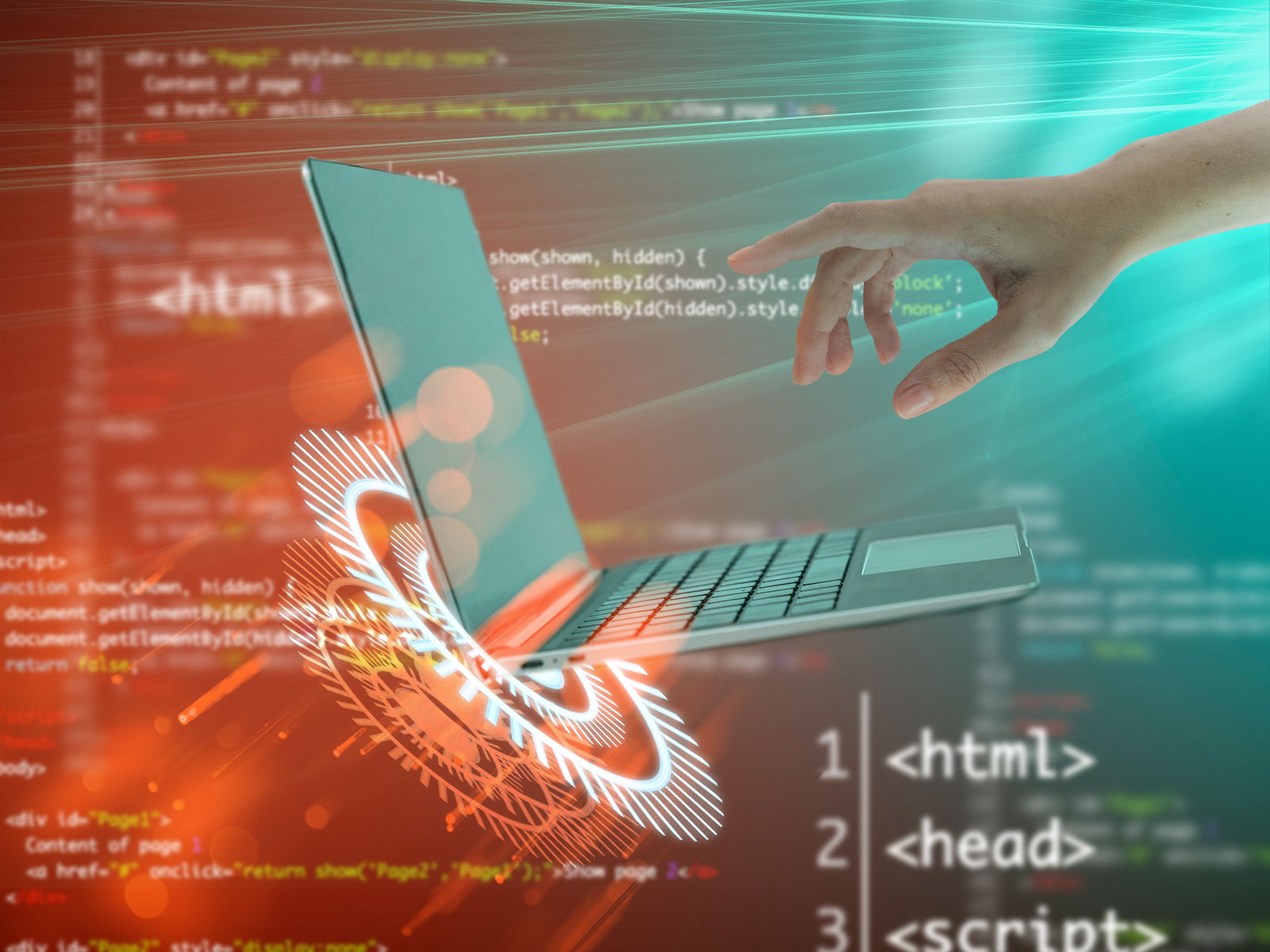
Embedded artificial intelligence: the new standard for personalized digital experiences
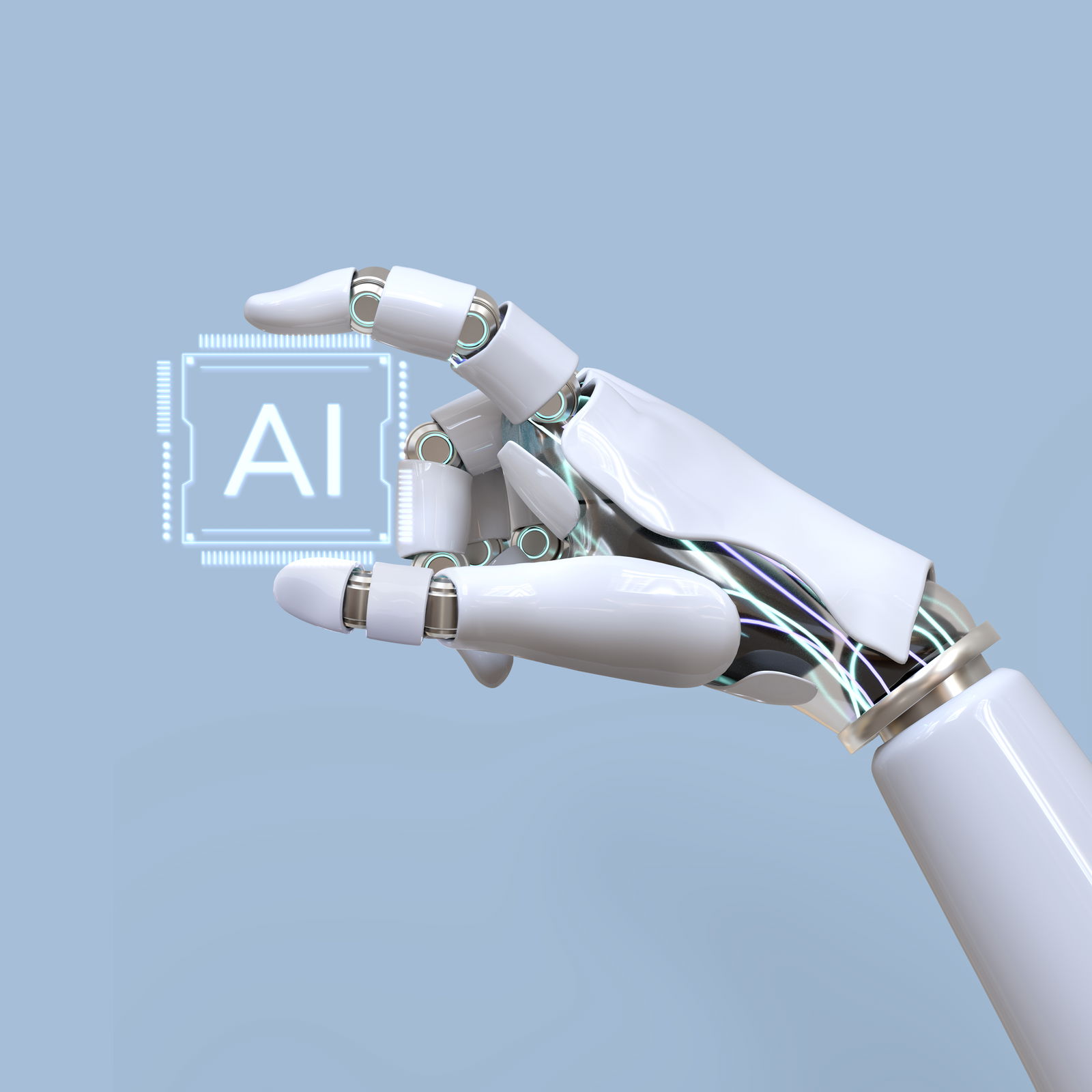